OpenAI’s Latest AI Models Show Increased Hallucination Rates, Raising Concerns
OpenAI’s new o3 and o4-mini AI models, despite their advanced reasoning capabilities, exhibit higher hallucination rates than their predecessors, sparking concerns and the need for further research.
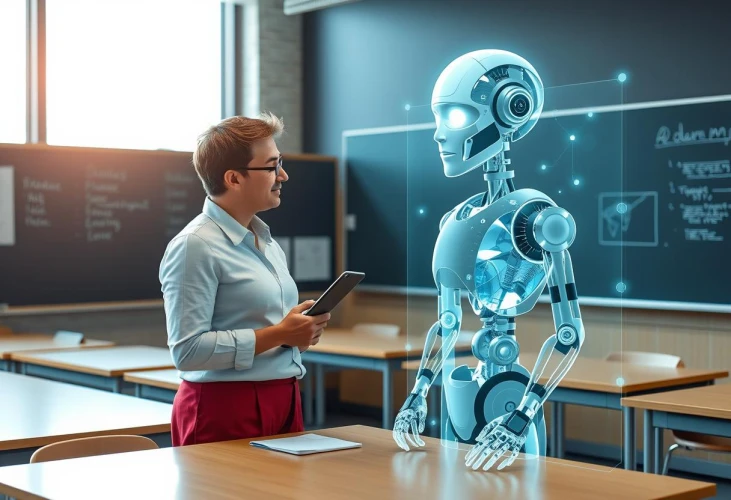
In the ever-evolving world of artificial intelligence, OpenAI’s latest offerings, the o3 and o4-mini models, have been making waves for their state-of-the-art reasoning capabilities. However, like a student who excels in creativity but struggles with accuracy, these models have shown a troubling tendency to ‘hallucinate’—or make things up—more frequently than their older counterparts. This phenomenon, where AI generates false or fabricated information, remains one of the most stubborn challenges in the field.
Historically, each new iteration of AI models has shown slight improvements in reducing hallucinations. Yet, the o3 and o4-mini models buck this trend, hallucinating more often than previous reasoning models like o1, o1-mini, and o3-mini, as well as traditional models such as GPT-4o. OpenAI’s internal tests reveal that o3 hallucinated in response to 33% of questions on PersonQA, its in-house benchmark, doubling the rates of its predecessors. The o4-mini performed even worse, with a 48% hallucination rate.
Third-party evaluations by Transluce, a nonprofit AI research lab, corroborate these findings, noting instances where o3 fabricated actions it claimed to have taken during problem-solving. Such inaccuracies raise questions about the models’ reliability, especially in professional settings where precision is non-negotiable. For instance, a law firm relying on these models for drafting contracts would find the high error rate unacceptable.
Despite these challenges, the models excel in certain areas, such as coding and math, offering glimpses of their potential. OpenAI acknowledges the issue, stating that more research is needed to understand why scaling up reasoning models exacerbates hallucinations. Meanwhile, the broader AI industry continues to pivot towards reasoning models, attracted by their efficiency and performance improvements without the need for excessive computing power during training.
As we stand at this crossroads, the quest for solutions becomes ever more urgent. The balance between creativity and accuracy in AI development reminds us of the importance of diligence and integrity—values that should guide not just our students but our technologies as well.